Pretraining Just Got Smarter: How Essential AI’s Research Redefines the Role of Reasoning in Model Development
Apr 25, 2025
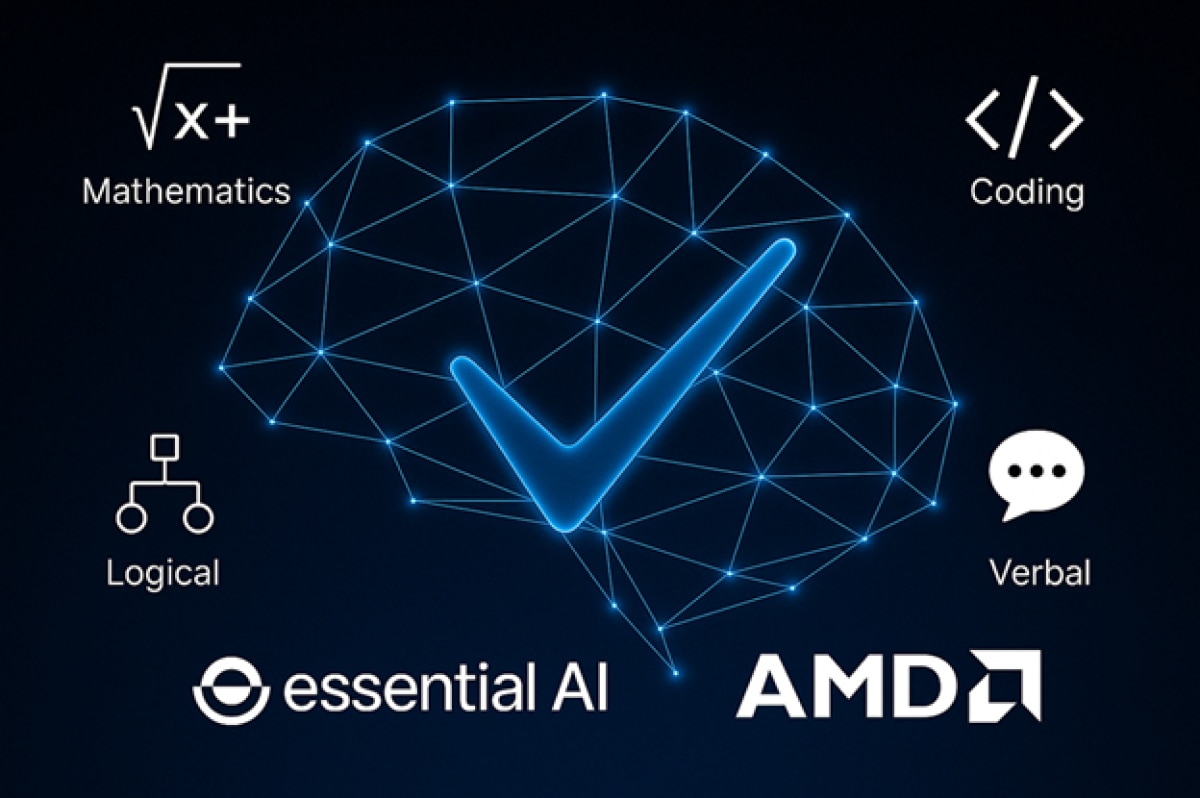
Introduction
Traditionally, reasoning in language models was thought to emerge during post-training, particularly through reinforcement learning or instruction tuning. But a groundbreaking new paper from Essential AI, Rethinking Reflection in Pre-Training, challenges that assumption—and shifts the conversation upstream.
Key Insight
Essential AI shows that reasoning and self-reflection capabilities can begin forming during pretraining, especially in relatively small models. Among the models studied, a 7B OLMo-2 model, trained on just 198 billion tokens, was shown to exhibit reflection across math, code, logical, and verbal tasks.
“We determine that models trained with very few training flops, like OLMo-2-7B trained with 198 billion tokens, demonstrate reflection across Mathematics, Code, Verbal, and Logical Reasoning. Furthermore, as more compute is spent on pre-training, these abilities further strengthen.”
This research highlights a critical takeaway: the more compute invested in pretraining, the stronger these reflective abilities become.
Why It Matters
- Efficiency: This work suggests reasoning doesn’t require massive instruction tuning pipelines—it can start forming earlier.
- Scalability: As organizations scale up pretraining compute, they may see even stronger capabilities emerge naturally, leading to lower inference time costs.
- Open Science: Essential AI made this discovery using openly available models and training it on openly licensed data—and published everything publicly.
Powered by AMD Instinct™ MI300X GPUs
Essential AI ran these experiments using a cluster of AMD Instinct™ MI300X GPUs, managed with Kubernetes, and serving models through vLLM and SGLang. Their results underscore the power of AMD infrastructure for cutting-edge research in LLM training and inference.
This collaboration represents what’s possible in the open ecosystem when research and hardware innovation align—without compromise.
Summary
Essential AI's discovery doesn’t just advance the field—it redefines the starting point for reasoning in language models. By showing that reflection can emerge early and scale with compute, they’ve opened new doors for efficient model development.
At AMD, we’re proud to support the open ecosystem to advance what is possible with AI.
Read the full paper: https://arxiv.org/abs/2504.04022
Follow the conversation: Ashish Vaswani on X | My post on LinkedIn and X