Enterprise AI is Becoming an End-to-End Infrastructure Evolution
Dec 16, 2024
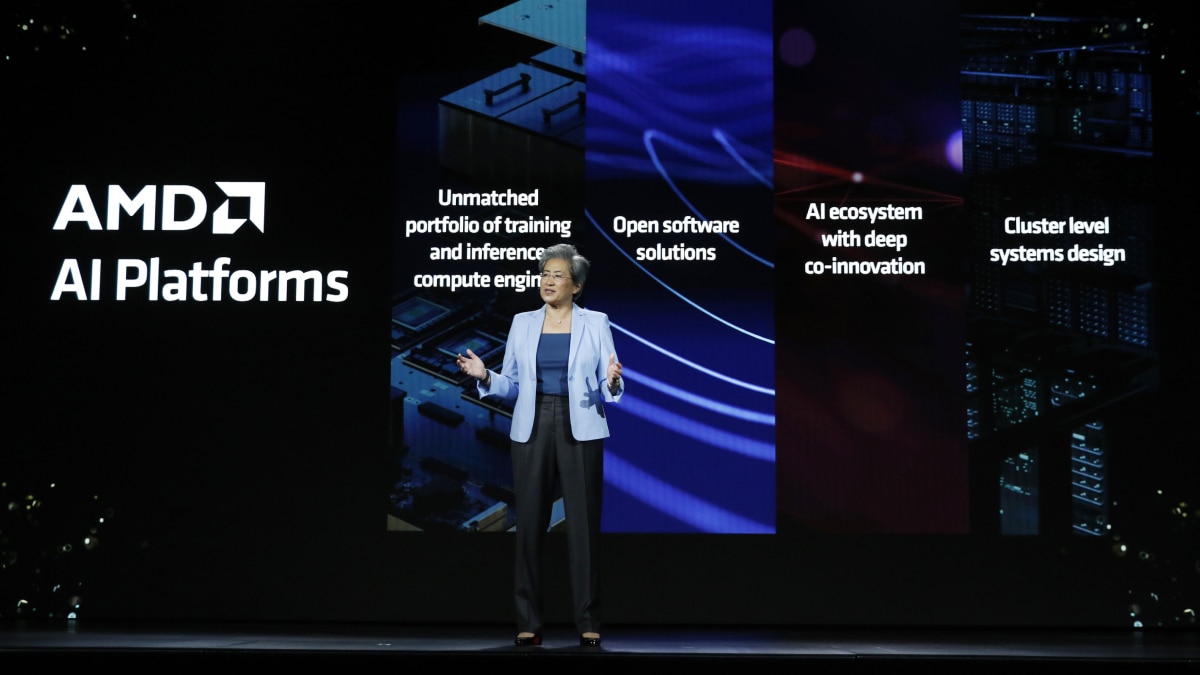
Artificial Intelligence (AI) is currently the biggest topic in enterprise technology. Customer facing applications, internal development tools, employee communications, and much more are being revolutionized with AI, and for each new use case the complexity and variety of the underlying infrastructure required to support this work grows.
But AI doesn’t have a one size fits all solution. Business leaders need to ensure the solutions they are implementing solve the unique AI requirements they need to stay competitive. CPUs, GPUs, networking products, and AI PCs are all key to successful AI infrastructure. AI, once considered a GPU-dependent, cluster-level workload is permeating all areas of the enterprise.
To make the most of these new AI opportunities, enterprise leaders need end-to-end AI solutions, from the data center, to the cloud, to the PCs their teams use every day. AMD is a technology leader, offering an unmatched data center portfolio. From new AI accelerators, to the recent launch of 5th Generation AMD EPYC™ processors, to networking products that can increase performance and efficiency at the system and environment levels, and even AI PCs for the enterprise – AMD offers a solution for every business need.
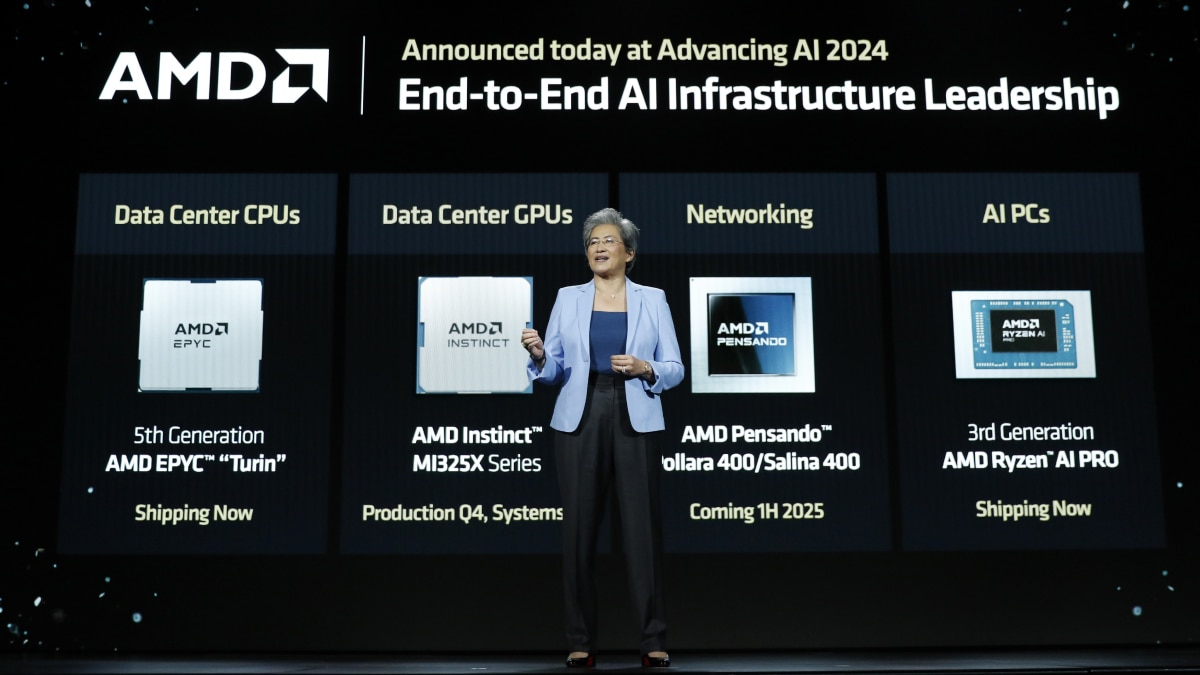
The Value of CPU-driven AI
It may be surprising to learn that broad classes of AI workloads run very efficiently on CPU-only server infrastructure. AI workloads—language models roughly 13 billion parameters and below, classical machine learning workloads such as image and fraud analysis or decision trees and recommendation systems offer strong performance on CPU-only systems. AMD EPYC 9005 Series processors are purpose-designed to excel when running these common AI and machine learning workloads.
In testing, AMD EPYC 9965 processors have a ~3.8x performance advantage over competitive top-of-stack processors when running TPCx-AI, an aggregate benchmark that represents end-to-end AI workloads1. Choosing AMD EPYC 9005 processors could be the optimal AI hardware solution for organizations running a wide range of smaller AI workloads.
For larger workloads that do rely on GPU acceleration, AMD EPYC 9005 still provides incredible value. Many organizations running legacy hardware could consolidate their data center footprint by modernizing to AMD EPYC 9005. In an AMD scenario comparing EPYC 9965 powered servers to legacy Intel Xeon 8280 based servers using integer performance, users can generate comparable performance output with up to a 7:1 consolidation ratio to help reduce TCO and make space for new, GPU accelerated equipment.2
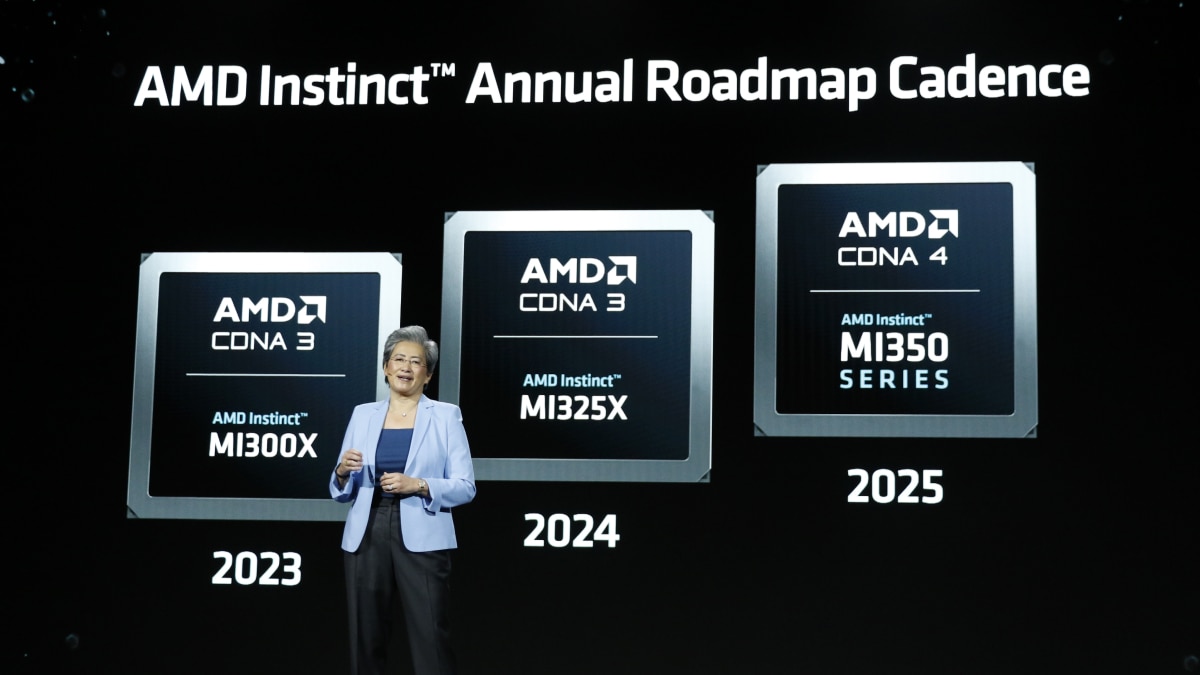
GPUs are Evolving Rapidly
GPU acceleration has always been a centerpiece of the AI infrastructure world, and that is not changing. Now, GPUs are evolving faster than ever, and the competition in the GPU space is heating up. The AMD Instinct™ MI325X GPU accelerator is the latest in a now yearly roadmap of AMD Instinct GPUs. This new accelerator, built with 3rd Gen AMD CDNA™ architecture, delivers incredible performance and efficiency for training and inference. Across several inference benchmarks, MI325X outperforms the competition by up to 40%3,4,5.
The speed of innovation for AMD Instinct products is also highlighted by the upcoming MI355x accelerator, on-track to launch in the second half of 2025. The MI350 series will feature next-generation AMD CDNA4 architecture, up to 288GB of memory, and support for FP4 and FP6 data types. MI355x will be followed by the MI400 series, planned for 2026 showcasing the continued focus on growing GPU performance.
AI is not the only space that benefits from these advancements in GPU performance. For example, high-performance computing systems like El Capitan, the most powerful supercomputer in the world as of November 2024, leverages AMD Instinct GPUs to run modeling and simulation capabilities essential to the NNSA’s nuclear stockpile stewardship directive, among other important work.
These hardware advancements are underpinned by continued improvements to ROCm™ open software, which features new algorithms, libraries, and broad platform support.
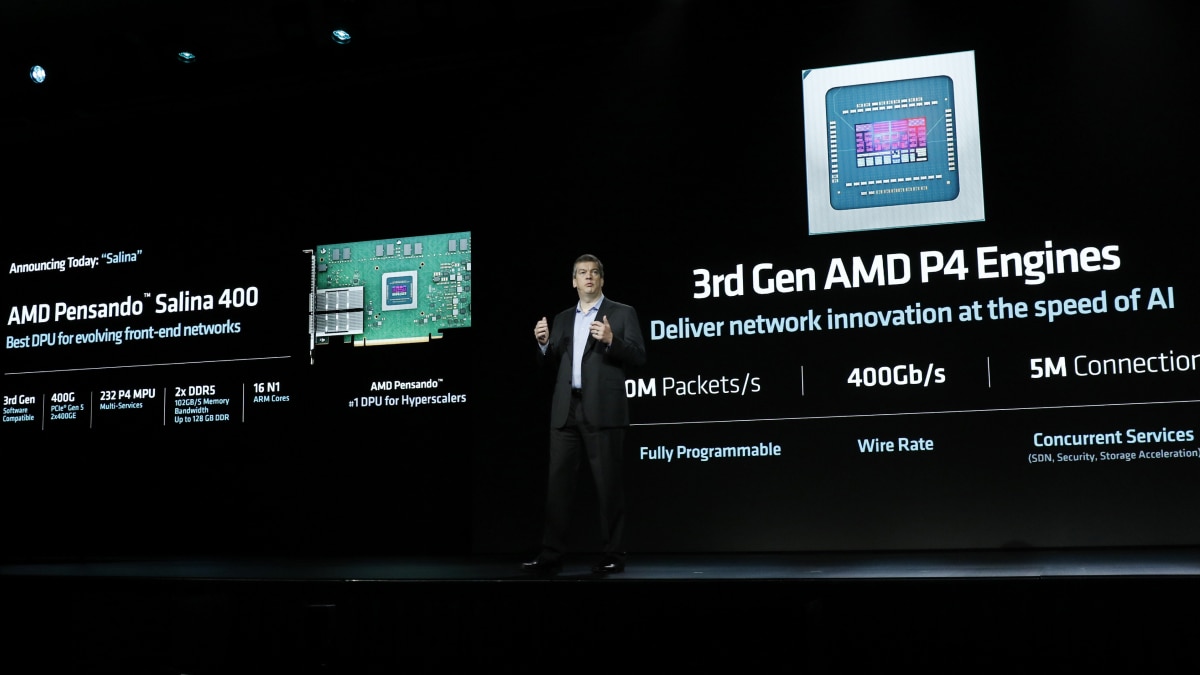
Networking is the Next Challenge for Large Scale AI
As AI-ready data centers grow and ecosystems expand, having a robust networking solution is critical to making the most of those investments in servers and accelerators. Without advanced networking solutions, individual GPUs, servers, and even clusters can be limited by an inability to transfer data quickly enough. In this scenario, even the most powerful GPU-accelerated servers would be bottlenecked by the network and lose overall performance.
To solve this, the industry needs newer, more powerful networking technology that can keep up with the speed of AI. That is why AMD is a member of the Ultra Ethernet Consortium, a group of industry leaders working to make Ethernet, the longtime standard for general purpose data center networking, the ideal fabric for AI networking.
Also, new networking components can improve the efficiency and performance of systems. The all-new AMD Pensando™ Pollara 400 NIC is changing the way we think about networking for AI. Designed to meet the unique demands of AI networking, the Pollara 400 NIC enables efficient, reliable, and high-speed data transfers. Meanwhile, the AMD Pensando Salina 400 DPU is designed to offload networking tasks from the CPU, helping improve performance for critical workloads.
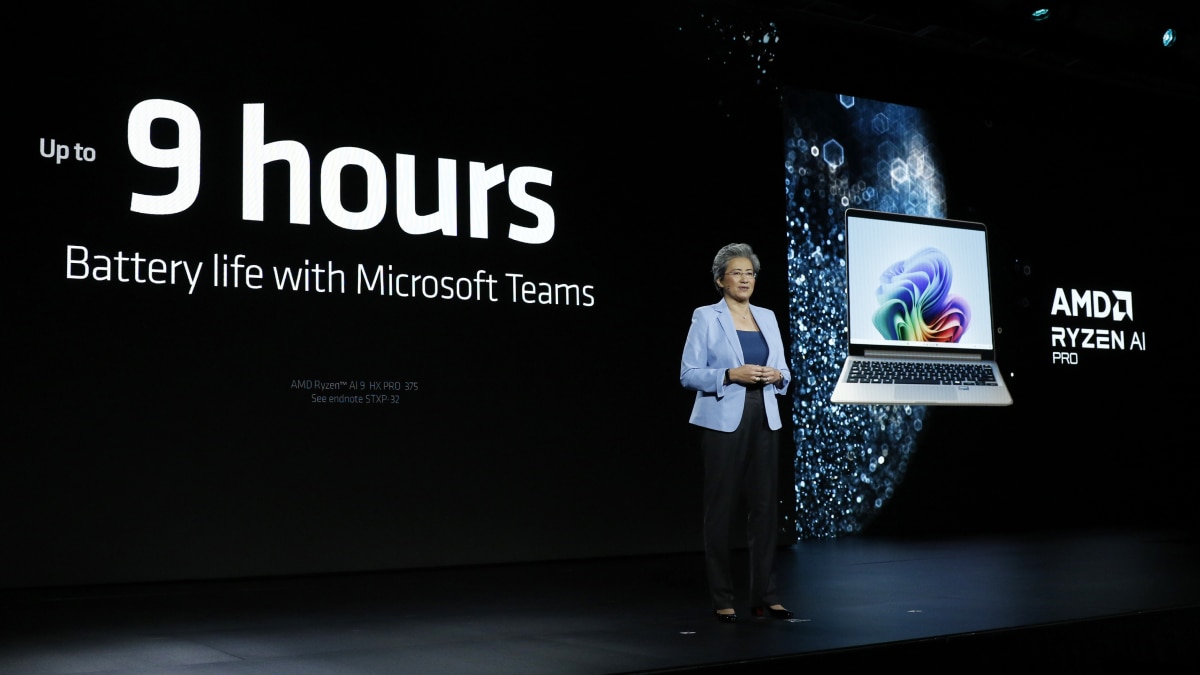
AI PCs are Coming to the Enterprise
Next generation AI PCs are already impacting the way we work. AI functionality on business PCs contribute to improved productivity, more immersive and functional collaboration, faster and more optimized creativity and production, and even AI assistance. All of this can happen locally on the device.
Ryzen™ AI PRO 300 series processors, the best lineup of commercial processors for Copilot+ laptops designed for enterprise users are here6. Its new NPU features over 50 TOPS of AI performance enabling a faster, more efficient AI PC experience.
The need for End-to-End AI Infrastructure
Building the infrastructure for AI-first enterprises is a complex challenge that is often unique to each organization and their specific goals in AI. Finding the right balance of new technologies across the data center, cloud computing, and enterprise AI PCs is critical to deploying successful AI applications and revolutionizing the enterprise.
That is why; to solve these complex AI challenges anywhere in the technology stack, AMD provides an end-to-end portfolio of CPUs, GPUs, Networking Products, and AI PCs that provide leadership AI performance and efficiency.
To learn even more about the AMD AI roadmap and all the latest innovations for enterprises and the data center watch the Advancing AI 2024 event replay here.
Resources
- 9xx5-012: TPCxAI @SF30 Multi-Instance 32C Instance Size throughput results based on AMD internal testing as of 09/05/2024 running multiple VM instances. The aggregate end-to-end AI throughput test is derived from the TPCx-AI benchmark and as such is not comparable to published TPCx-AI results, as the end-to-end AI throughput test results do not comply with the TPCx-AI Specification.
2P AMD EPYC 9965 (384 Total Cores), 12 32C instances, NPS1, 1.5TB 24x64GB DDR5-6400 (at 6000 MT/s), 1DPC, 1.0 Gbps NetXtreme BCM5720 Gigabit Ethernet PCIe, 3.5 TB Samsung MZWLO3T8HCLS-00A07 NVMe®, Ubuntu® 22.04.4 LTS, 6.8.0-40-generic (tuned-adm profile throughput-performance, ulimit -l 198096812, ulimit -n 1024, ulimit -s 8192), BIOS RVOT1000C (SMT=off, Determinism=Power, Turbo Boost=Enabled)
2P AMD EPYC 9755 (256 Total Cores), 8 32C instances, NPS1, 1.5TB 24x64GB DDR5-6400 (at 6000 MT/s), 1DPC, 1.0 Gbps NetXtreme BCM5720 Gigabit Ethernet PCIe, 3.5 TB Samsung MZWLO3T8HCLS-00A07 NVMe®, Ubuntu 22.04.4 LTS, 6.8.0-40-generic (tuned-adm profile throughput-performance, ulimit -l 198096812, ulimit -n 1024, ulimit -s 8192), BIOS RVOT0090F (SMT=off, Determinism=Power, Turbo Boost=Enabled)
2P AMD EPYC 9654 (192 Total cores) 6 32C instances, NPS1, 1.5TB 24x64GB DDR5-4800, 1DPC, 2 x 1.92 TB Samsung MZQL21T9HCJR-00A07 NVMe, Ubuntu 22.04.3 LTS, BIOS 1006C (SMT=off, Determinism=Power)
Versus 2P Xeon Platinum 8592+ (128 Total Cores), 4 32C instances, AMX On, 1TB 16x64GB DDR5-5600, 1DPC, 1.0 Gbps NetXtreme BCM5719 Gigabit Ethernet PCIe, 3.84 TB KIOXIA KCMYXRUG3T84 NVMe, , Ubuntu 22.04.4 LTS, 6.5.0-35 generic (tuned-adm profile throughput-performance, ulimit -l 132065548, ulimit -n 1024, ulimit -s 8192), BIOS ESE122V (SMT=off, Determinism=Power, Turbo Boost = Enabled)
Results:
CPU Median Relative Generational
Turin 192C, 12 Inst 6067.531 3.775 2.278
Turin 128C, 8 Inst 4091.85 2.546 1.536
Genoa 96C, 6 Inst 2663.14 1.657 1
EMR 64C, 4 Inst 1607.417 1 NA
Results may vary due to factors including system configurations, software versions and BIOS settings. TPC, TPC Benchmark and TPC-C are trademarks of the Transaction Processing Performance Council. - 9xx5TCO-001A: This scenario contains many assumptions and estimates and, while based on AMD internal research and best approximations, should be considered an example for information purposes only, and not used as a basis for decision making over actual testing. The AMD Server & Greenhouse Gas Emissions TCO (total cost of ownership) Estimator Tool - version 1.12, compares the selected AMD EPYC™ and Intel® Xeon® CPU based server solutions required to deliver a TOTAL_PERFORMANCE of 39100 units of SPECrate2017_int_base performance as of October 3, 2024. This scenario compares a legacy 2P Intel Xeon 28 core Platinum_8280 based server with a score of 391 versus 2P EPYC 9965 (192C) powered server with an score of 3030 (https://spec.org/cpu2017/results/res2024q3/cpu2017-20240923-44833.pdf) along with a comparison upgrade to a 2P Intel Xeon Platinum 8592+ (64C) based server with a score of 1130 (https://spec.org/cpu2017/results/res2024q3/cpu2017-20240701-43948.pdf). Actual SPECrate®2017_int_base score for 2P EPYC 9965 will vary based on OEM publications.
Environmental impact estimates made leveraging this data, using the Country / Region specific electricity factors from the 2024 International Country Specific Electricity Factors 10 – July 2024 , and the United States Environmental Protection Agency 'Greenhouse Gas Equivalencies Calculator'.
For additional details, see https://www.amd.com/en/legal/claims/epyc.html#q=epyc5#9xx5TCO-001A - MI325-004: Based on testing completed on 9/28/2024 by AMD performance lab measuring text generated throughput for Mixtral-8x7B model using FP16 datatype. Test was performed using input length of 128 tokens and an output length of 4096 tokens for the following configurations of AMD Instinct™ MI325X GPU accelerator and NVIDIA H200 SXM GPU accelerator.
1x MI325X at 1000W with vLLM performance Vs.1x H200 at 700W with TensorRT-LLM v0.13
Configurations:
AMD Instinct™ MI325X reference platform: 1x AMD Ryzen™ 9 7950X CPU, 1x AMD Instinct MI325X (256GiB, 1000W) GPU, Ubuntu® 22.04, and ROCm™ 6.3 pre-release
Vs
NVIDIA H200 HGX platform: Supermicro SuperServer with 2x Intel Xeon® Platinum 8468 Processors, 8x Nvidia H200 (140GB, 700W) GPUs [only 1 GPU was used in this test], Ubuntu 22.04) CUDA® 12.6
Server manufacturers may vary configurations, yielding different results. Performance may vary based on use of latest drivers and optimizations. - MI325-005: "Based on testing completed on 9/28/2024 by AMD performance lab measuring overall latency for Mistral-7B model using FP16 datatype. Test was performed using input length of 128 tokens and an output length of 128 tokens for the following configurations of AMD Instinct™ MI325X GPU accelerator and NVIDIA H200 SXM GPU accelerator.
1x MI325X at 1000W with vLLM performance: 0.637 sec (latency in seconds)
Vs.
1x H200 at 700W with TensorRT-LLM: 0.811 sec (latency in seconds)
Configurations:
AMD Instinct™ MI325X reference platform:
1x AMD Ryzen™ 9 7950X 16-Core Processor CPU, 1x AMD Instinct MI325X (256GiB, 1000W) GPU,
Ubuntu® 22.04, and ROCm™ 6.3 pre-release
Vs
NVIDIA H200 HGX platform:
Supermicro SuperServer with 2x Intel Xeon® Platinum 8468 Processors, 8x Nvidia H200 (140GB,700W) GPUs [only 1 GPU was used in this test], Ubuntu 22.04), CUDA 12.6
Server manufacturers may vary configurations, yielding different results. Performance may vary based on use of latest drivers and optimizations. MI325-005" - MI325-006: "Based on testing completed on 9/28/2024 by AMD performance lab measuring overall latency for LLaMA 3.1-70B model using FP8 datatype. Test was performed using input length of 2048 tokens and an output length of 2048 tokens for the following configurations of AMD Instinct™ MI325X GPU accelerator and NVIDIA H200 SXM GPU accelerator.
1x MI325X at 1000W with vLLM performance: 48.025 sec (latency in seconds)
Vs.
1x H200 at 700W with TensorRT-LLM v 0.13: 56.310 sec (latency in seconds)
Configurations:
AMD Instinct™ MI325X reference platform:
1x AMD Ryzen™ 9 7950X 16-Core Processor CPU, 1x AMD Instinct MI325X (256GiB, 1000W) GPU, Ubuntu® 22.04, and ROCm™ 6.3 pre-release
Vs
NVIDIA H200 HGX platform:
Supermicro SuperServer with 2x Intel Xeon® Platinum 8468 Processors, 8x Nvidia H200 (140GB, 700W) GPUs, Ubuntu 22.04), CUDA 12.6
Server manufacturers may vary configurations, yielding different results. Performance may vary based on use of latest drivers and optimizations. MI325-006" - STXP-04a: Based on product specifications and competitive products announced as of Oct 2024 and testing as of Sept 2024 by AMD performance labs using the following systems: HP EliteBook X G1a with AMD Ryzen AI 9 HX PRO 375 processor @23W, Radeon 880M graphics, 32GB of RAM, 512GB SSD, VBS=ON, Windows 11 PRO; Dell Latitude 7450 with Intel Core Ultra 7 165U processor @15W (vPro enabled), Intel Iris Xe Graphics, VBS=ON, 32GB RAM, 512GB NVMe SSD, Microsoft Windows 11 Professional; Dell Latitude 7450 with Intel Core Ultra 7 165H processor @28W (vPro enabled), Intel Iris Xe Graphics, VBS=ON, 16GB RAM, 512GB NVMe SSD, Microsoft Windows 11 Pro. All systems were tested in Best Performance Mode. STXP-04a.