[How-To] Automatic1111 Stable Diffusion WebUI with DirectML Extension on AMD GPUs
Nov 30, 2023
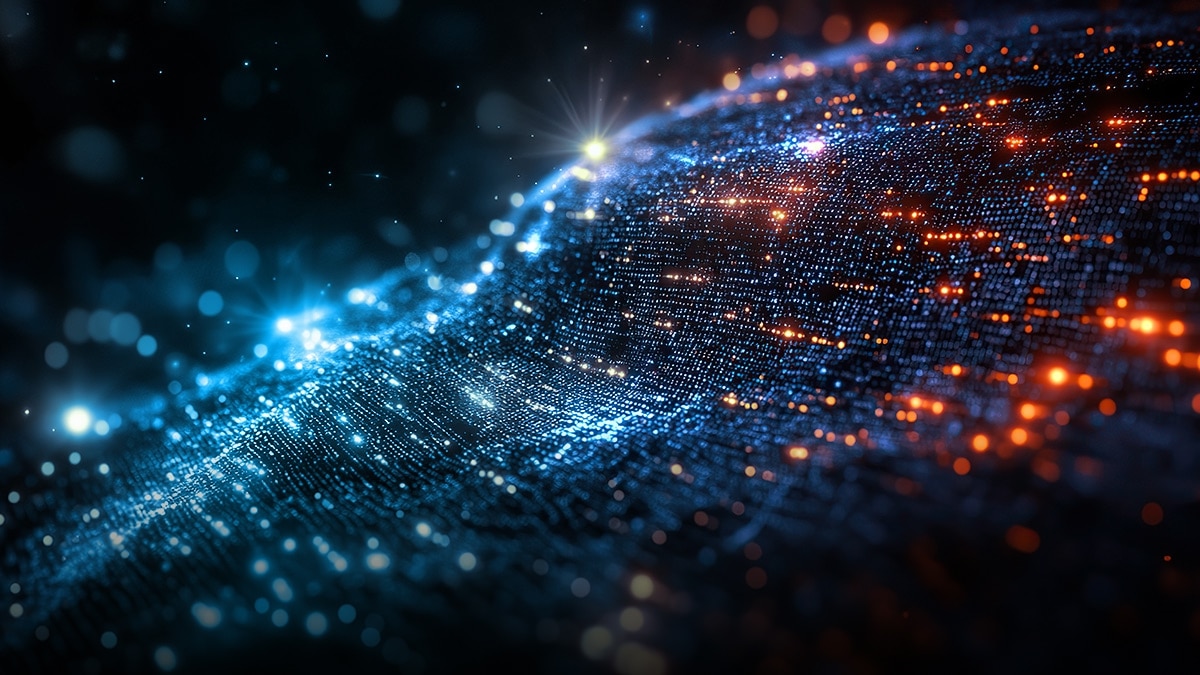
Prepared byHisham Chowdhury (AMD),Sonbol Yazdanbakhsh (AMD), Justin Stoecker (Microsoft), and Anirban Roy (Microsoft)
Microsoft and AMD continue to collaborate enabling and accelerating AI workloads across AMD GPUs on Windows platforms. We published an earlier article about accelerating Stable Diffusion on AMD GPUs using Automatic1111 DirectML fork.
Now we are happy to share that with ‘Automatic1111 DirectML extension’ preview from Microsoft, you can run Stable Diffusion 1.5 with base Automatic1111 with similar upside across AMD GPUs mentioned in our previous post
Fig 1: up to 12X faster Inference on AMD Radeon™ RX 7900 XTX GPUs compared to non ONNXruntime default Automatic1111 path
Microsoft and AMD engineering teams worked closely to optimize Stable Diffusion to run on AMD GPUs accelerated via Microsoft DirectML platform API and AMD device drivers. AMD device driver resident ML acceleration layers utilize AMD Matrix Processing Cores via wavemma intrinsics to accelerate DirectML based ML workloads including Stable Diffusion, Llama2 and others.
Fig 2:OnnxRuntime-DirectML on AMD GPUs
- Installed Git (Git for Windows)
- Installed Anaconda/Miniconda (Miniconda for Windows)
- Ensure Anaconda/Miniconda directory is added to PATH
- Platform having AMD Graphics Processing Units (GPU)
- Driver: AMD Software: Adrenalin Edition™ 23.11.1 or newer(https://www.amd.com/en/support)
Olive is a Python tool that can be used to convert, optimize, quantize, and auto-tune models for optimal inference performance with ONNX Runtime execution providers like DirectML. Olive greatly simplifies model processing by providing a single toolchain to compose optimization techniques, which is especially important with more complex models like Stable Diffusion that are sensitive to the ordering of optimization techniques. The DirectML sample for Stable Diffusion applies the following techniques:
- Model conversion:translates the base models from PyTorch to ONNX.
- Transformer graph optimization:fuses subgraphs into multi-head attention operators and eliminating inefficient from conversion.
- Quantization:converts most layers from FP32 to FP16 to reduce the model's GPU memory footprint and improve performance.
Combined, the above optimizations enable DirectML to leverage AMD GPUs for greatly improved performance when performing inference with transformer models like Stable Diffusion.
Follow these steps to enable DirectML extension on Automatic1111 WebUI and run with Olive optimized models on your AMD GPUs:
**only Stable Diffusion 1.5 is supported with this extension currently
**generate Olive optimized models using our previous post or Microsoft Olive instructions when using the DirectML extension
**not tested with multiple extensions enabled at the same time
Open Anaconda Terminal
- conda create --name automatic_dmlplugin python=3.10.6
- conda activate automatic_dmlplugin
- git clone https://github.com/AUTOMATIC1111/stable-diffusion-webui
- cd stable-diffusion-webui
- webui.bat --lowvram --precision full --no-half --skip-torch-cuda-test
Open the Extensions tab
- go to Install from URL and paste in this URL: https://github.com/microsoft/Stable-Diffusion-WebUI-DirectML
- Click ‘install’
Copy the Unet model optimized by Olive to models\Unet-dml folder
- example \models\optimized\runwayml\stable-diffusion-v1-5\unet\model.onnx -> stable-diffusion-webui\models\Unet-dml\model.onnx folder.
Return to the Settings Menu on the WebUI interface
- Settings → User Interface → Quick Settings List, add sd_unet
- Apply settings, Reload UI
Navigate to the "Txt2img" tab of the WebUI Interface
- Select the DML Unet model from the sd_unet dropdown
Run your inference!
